SMART
SERVICING FOR ROBOTS - Those looking for a future proofed infrastructure for
renewably sourced energy for electric vehicles, may want to consider the new
breed of self driving passenger cars, taxis, and commercial trucks that will benefit from automated
recharging if they are to be fully autonomous in operation - most especially
unmanned robotic taxis and freight vehicles.
Robotaxis
hold the potential of taking city transport to a new level, maybe even
displacing scheduled bus services altogether. But only if there are energy
service facilities to keep this with the new breed of automobiles that
start, steer and stop automatically as the situation demands, supplied with
energy.
Any
autonomous vehicles assumes excellent navigation
skills and ultra-reliable robotics
onboard, operated by computers and graphics cards with previously unheard of
number crunching abilities, and sensors that can see with the eyes of an
eagle.
The
majority of road accidents are caused by human error, not mechanical
failure. It stands to reason that
taking people out of the equation could take out the majority of dings, but, the general public are not fully convinced of the safety credentials of driverless
cars. This was the same when horseless carriages where introduced and
parliament decided they would only be safe with a man walking ahead carrying
a red
flag - a cooling off period for enthusiastic motorists perhaps. But
governments all around the world had to concede the point despite the
stagecoach lobby. Shoveling horse dung from the city streets soon gave way
to chugging and belching exhausts - and now we have cured that hiccup with
zero emission electric vehicles about to displace diesel particulates.
An
area that curiosity is getting the better of most of us is a vehicle with an
empty driver's seat. These machines are at the moment something to wonder
at, but like the first mobile phones, the size of 'bricks,' that only the
wealthy lugged around from Mansion to Bentley, smartphones are now pocket
sized and an essential part of every day life, affordably.
PONY.ai - Self driving trucks for freeway haulage logistics is all the
rage at the moment for investors looking for the latest digital gold rush.
Kodiak joins Waymo, Aurora,
TuSimple
and AutoX
as they look for the elusive holy grail of AI on the road, somewhat blowing
wildcard Tesla
into the weeds. You can read more about these companies and the media
coverage, and reviews, on this site. Strangely, even with the hydrogen
revolution taking place all over the world, there is as yet no energy
vending system for these autonomous vehicles. It's competition as usual, in
a world calling for collaboration.
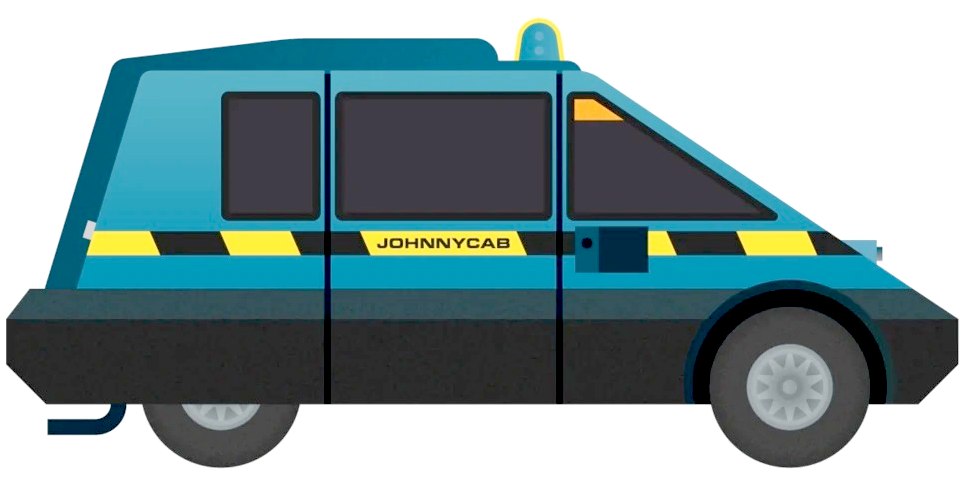
“YOU'RE IN A JOHNNY CAB”
- What is less of a struggle, by today’s standards, is the concept and use of self-driving cars as a technology prediction in Total Recall. As far as the accuracy of that prediction, it’s nearly spot-on. The film is set far enough into the future that it’s reasonable to assume most of the technical kinks would have been worked out. The other significant thing to notice is where in Total Recall they use self-driving technology: in taxi cabs.
The cab company in Total Recall is called Johnny
Cab. They are all standard in look, feel, and user interface. In this case, it’s a voice recognition controlled robot driver that always refers to itself as “Johnny Cab.” Said robot driver is smarmy, chatty, and every inch a creepy puppet-like thing with wheeling mannerisms and snide eye rolls. It’s a trope put in more for comic effect than anything else.
The reason for this near inevitability isn’t necessarily the technology we have at our disposal, but more how companies function. Let’s face it: companies exist mainly to make money – as much money as they can. And (not to be too brutal about it) employees cost a lot. There are salaries, benefits, labor relations, workmen’s comp and such. All of that gets taken out of the “cost” column and neatly deposited in the “profits” column if you can cut out the human element.
Trucking companies and cab companies, and even quasi-cab companies like Uber, are betting heavily on an autonomous future. I wouldn’t want to be a long haul trucker 10 or 15 years from now. Even though some sources say jobs won’t be affected, it’s hard to imagine just taking that at face value if you are a life-long truck driver. On the other hand, I wouldn’t want to be the trucking or tech company trying to make this happen, because truck drivers are an essential part of our everyday economy.
DEBUGGER.MEDIUM.COM - 4 FEBRUARY 2020
- An Automated Vehicle Expert Explains How Close We Are to Robotaxis
Humans are notoriously bad at estimating how long it will take to do something that hasn’t been done before, particularly when it comes to technology that doesn’t exist yet. Amara’s Law sums it up most succinctly: “We overestimate the effect of technology in the short-term and underestimate the effect in the long run.” The impact of
Automated Driving Systems (ADS) is a prime example. At some point, automated vehicles (AVs), including
robotaxis, will likely become a primary means of transporting people and goods, but a lot has to happen between now and then.
AVs are an idea almost as old as electric and hybrid vehicles. Ferdinand
Porsche built the first hybrid car, the 1901 Lohner-Porsche. But it took another century for the idea to become mainstream while the technologies matured. Even the power-split hybrid architecture popularized by
Toyota with the Prius when it debuted in 1997 was patented nearly three decades earlier. Still, we lacked the electronic control systems and batteries to make it useful.
One of the earliest promotional examples of an AV was a 1956 General Motors film featuring the Firebird II concept. But conceiving an idea and making it commercially viable are very different things. It wasn’t until the DARPA Grand Challenge between 2003 and 2007 that anyone was able to demonstrate the first hints of truly workable AVs, and even those vehicles were very much science projects.
My first ride in an AV at the 2008 CES in Las Vegas was in the Carnegie Mellon University Chevrolet Tahoe that had won the Urban Challenge event a few months earlier. That vehicle successfully navigated a course set up in what was then a parking lot at the Las Vegas Convention Center.
Humans are notoriously bad at estimating how long it will take to do something that hasn’t been done before, particularly when it comes to technology that doesn’t exist yet. Amara’s Law sums it up most succinctly: “We overestimate the effect of technology in the short-term and underestimate the effect in the long run.” The impact of automated driving systems (ADS) is a prime example. At some point, automated vehicles (AVs), including robotaxis, will likely become a primary means of transporting people and goods, but a lot has to happen between now and then.
Three years later, GM was back at CES, demonstrating the Electric Networked-Vehicle (EN-V) concepts that debuted at the Shanghai World Expo prior year. The mastermind behind the EN-V automated pods, Chris Borroni-Bird, PhD, projected that something like the EN-V could be in wide use by 2020. Over the past decade, we’ve seen others, including former Uber CEO Travis Kalanick, predicting that computers would replace human drivers as soon as 2018.
Needless to say, in early 2021, unless you happen to live in certain very limited portions of the Phoenix suburbs, you can’t just hail a ride and have it show up without a human sitting behind the steering wheel. Other cities like Las Vegas, Singapore, and Shanghai have robotaxi pilots, but they all still have human safety operators. What will it take to get from where we are to having robotaxis accounting for 95% of passenger miles as Tony Seba predicted in 2017?
AUTOX
- This is one of the growing fleet of AutoX robotaxis currently operating in
China. Along with Waymo in the US, the advent of robotic cars is creating
quite a stir in media articles as you can see from the coverage featured on
this website, and how delightful it will be when buses and our personal
vehicles, automatically take us to our destinations, free of speeding
tickets - and safer - also with less harm to the environment as tire wear
will be reduced.
HOW
DO WE GET OUR ROBOTAXIS?
Going from a science project like the DARPA Grand Challenge to safe, reliable, and commercially viable AVs is a monumentally difficult challenge. That Tahoe I road in 13 years ago was packed with computer servers and bristling with sensors that were state of the art for the time, but nowhere near ready for volume production. The first generation Velodyne lidar sensors cost $80,000 and weren’t designed for the rigors of daily driving. Many hurdles must be overcome before you can step out to the sidewalk to get your “Johnny Cab.” First up is maturing the foundational technology of ADS. In its most simple form, you can think of an ADS as four steps — perception, prediction, planning, and control. This is exactly what our brain does when we drive.
Perception is the process of using electronic sensors to survey the environment around a vehicle and then processing the raw bits to get a semantic understanding of that environment. Essentially, look around and recognize and distinguish between other vehicles, motorcycles, bicycles, animals, traffic signals, debris, and of course, pedestrians.
Once the system knows what’s out there, it has to predict what each of those targets will do in the next few seconds. If there is a pedestrian on the sidewalk, the ADS has to make a decision if that person is likely to turn and cross the street. Will that car coming toward me turn left across my current path? Will that motorcycle decide to move from the center of the adjacent lane to split the lanes?
Once perception and prediction are complete, the ADS has to plot a safe path through the environment. The system needs an understanding of the rules of the road in the place where it is operating. It needs to know if it should be driving on the left or right side of the road. Can it make a turn against a red light? Can it cross the double yellow line to go around a double-parked delivery van?
Finally, once all the decisions have been made, it can send signals to the vehicle’s propulsion, braking, and steering actuators to execute the plan. It also needs to activate relevant indicators such as turn signals, light boards, or other signals to indicate its intent to other road users.
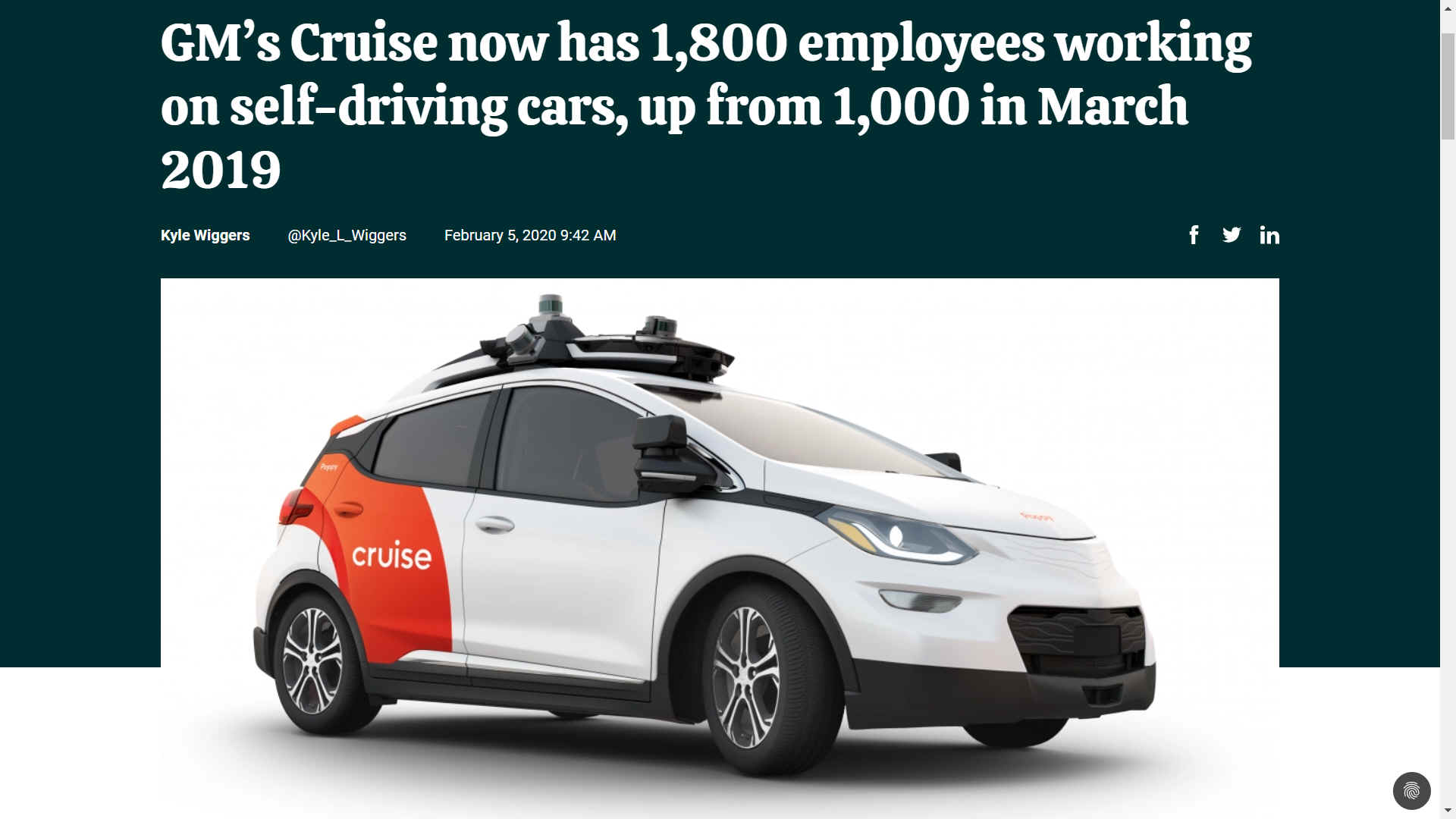
VENTURE
BEAT 2020 - During GM’s investor day conference in New York this morning, Dan Ammann, CEO of the automaker’s self-driving subsidiary Cruise Automation, gave a glimpse at the progress it’s made toward a fully self-driving vehicle fleet. He said that Cruise’s engineers, of which there are now 1,800 (up from 1,000 in March 2019), have over the past four years helped improve autonomous driving performance by a factor of well over 1,000. Concretely, they’ve reduced the amount of time between major software updates by 98% while cutting the time it takes to train the AI underpinning its cars by 80%, such that major new firmware rolls out up 45 times more frequently than before (as often as twice weekly).
SEEING
THE WORLD
In the DARPA days and even until relatively recently, the focus of ADS developers was determining the right mix of sensors to get the necessary perception performance. Most have concluded that at least three distinct types of sensing modalities will be necessary for an ADS to perform at what is considered level 4 (L4). An L4 system is capable of operating the vehicle without human supervision or interaction in an operational design domain (ODD). An ODD can be any arbitrary constraints such as location, weather conditions, time of day, or any other limitation.
Each of the various types of sensor has distinct strengths and weaknesses, with some overlap of capabilities. Cameras, specifically red-green-blue (RGB) cameras that capture light in the visible spectrum are useful for classifying the targets around the vehicle. Various types of machine vision algorithms can pick out and distinguish between vehicles, pedestrians, animals, debris, and traffic signals. This area in particular is where artificial intelligence techniques like deep neural networks have had the biggest impact on performance. RGB cameras are inexpensive but they can struggle in low light conditions or in fog, snow, or rain. It’s also difficult to accurately measure the distance and trajectory of the target from two-dimensional images although multiple cameras fused together can provide some degree of depth information.
Radar is also common today in Advanced Driver-Assistance Systems (ADAS) and relatively inexpensive. It’s great for detecting metal objects and is largely impervious to the obstructions that mess with camera imagery. Radar can accurately measure distance and provide instantaneous speed measurements of the detected targets. But current generation automotive radar has relatively few channels and low resolution so it’s difficult to distinguish if a stationary object is a fire truck stopped on the shoulder or an overpass. Next-generation imaging radars provide much higher resolution but at a higher cost.
Lidar uses lasers to scan the surrounding environment and return a point cloud of reflected light pulses with highly accurate distance measurements. The currently more common time-of-flight lidars use pulsed laser beams and calculate the difference between subsequent pulses to determine the speed of the object. Newer frequency modulated continuous wave (FMCW) lidars use the Doppler effect to measure instantaneous speed in the same way as radar does with radio frequencies.
Lidar first came into automotive use during the DARPA Grand Challenge, but most sensors until now have been far too expensive and too delicate to withstand long-term use on vehicles that are subject to temperature, humidity, and vibration extremes. However, new lidar designs are now coming to market that cost $500–$1,000 rather than tens of thousands of dollars, and sub-$100 sensors should be available in the next three to five years.
Adding lidar and radar data to the camera images is also essential to reliably predicting where other road users are going. Without detailed positioning data of those road users, it’s nearly impossible to accurately determine the heading of those targets. A 2019 study conducted by Scale A.I. using sensor data from the nuScenes data set released by Aptiv showed the difficulty in measuring trajectory from 2D camera images. When adding in the lidar data, the measurements became much more accurate. Even small errors in the angular position of another vehicle can ruin the prediction of what that vehicle is about to do.
Like human drivers, robotaxis also need to look inward and be self-aware. In the absence of a human driver who will know when passengers leave something behind, smoke, or get ill in the vehicle, a robotaxi will need sensors. This may include smoke detectors, cameras for authentication and identifying if a passenger is in distress, and possibly even radar. Among other things, the sensors can determine if the vehicle needs to head back to a depot for cleaning or service.
Riders will also need to interact with the vehicle. In a cab or ride-hail vehicle, passengers can ask questions about the area or change a destination. In a robotaxi, touch screens will likely be present, some sort of digital voice assistant would also be helpful. Since riders may not be familiar with the syntax specific to any particular vehicle, a system that can handle natural language voice processing and provide a conversational interface would be very helpful, but it also requires significant computing capabilities.
COMPUTING WHAT IT ALL MEANS
The combination of these and other sensors can help create a highly accurate model of the environment around the vehicle. The human brain is shockingly good at the task of classifying what our eyes see. We’ve evolved over millions of years to be able to quickly distinguish that which might be dangerous from what can nurture us. But detecting speed and distance is more challenging. Meshing these different modalities can create a picture far beyond what the brain can do. But it requires an enormous amount of computing power.
In the DARPA Challenge days, the Carnegie Mellon Tahoe had 10 blade servers with Intel Core Duo processors that combined for an output of somewhere around 1.8 billion operations per second and filled the cargo area of the SUV. By the early 2010s, most developers had added in graphics processors that were much better suited to processing A.I. tasks thanks to their highly parallel architecture. Today, there are chips like the
Nvidia Xavier that offer 30 trillion operations per second (TOPS) while consuming far less power. But even that is now acknowledged to be insufficient for a robotaxi so Xavier is being used for L2 assist applications.
Next-generation chips like the Nvidia Orin, Qualcomm Snapdragon Ride, and Mobileye EyeQ5 and EyeQ6 are pushing performance into the hundreds of TOPS. Recently, Chinese automakers Nio and SAIC each announced upcoming vehicles using a compute platform with four Orin chips that compute at over 1,000 TOPS.
REDUNDANCY
& DIVERSITY
In current vehicles being driven by humans, the electronic assist systems typically aren’t built with redundancy. They do have diagnostic capabilities to detect a failure of hardware or software but they are designed to be fail-safe. That is they detect a fault, alert the driver, and disable the feature. The human driver is the redundant system. If anti-lock brakes fail, the human has to modulate the brake pedal. If the steering assist stops working, the human puts more effort into the wheel.
But what happens when there is no human driver or no controls for a human occupant to take over? AVs must have redundant and diverse systems that provide fail-operational capability that can bring the vehicle to a safe stop, preferably out of traffic. Diversity means that not only are backup systems required, but they need to be architecturally different. If the problem is caused by a software flaw or design flaw in a processor, two identical systems will make the same mistake and the system won’t be fail-operational. It may not even detect the flaw.
To ensure safety, autonomous vehicles require distinct compute platforms running different software. AVs require different sensing types so that when vision software for a camera doesn’t accurately measure the distance or direction of a cyclist, radar or lidar should. One of the approaches to diverse software is to have the primary code perceive the environment and predict what targets will do based on
A.I. algorithms and feed that information to the path planner. In parallel, the sensor data can be fed to a deterministic classical algorithm that is designed to make sure the vehicle doesn’t cause a crash.
Two implementations of this concept are Nvidia’s Safety Force Field (SFF) and Mobileye’s Responsibility Sensitive Safety (RSS) model. Both of these act as a sanity check on the primary system and can help to bring the vehicle to safety if they detect a problem.
In addition to the compute platform and software, a robotaxi (or any other L4 AV) also requires redundancy for the actuation systems that actually get the commands and make the vehicle go, stop, and steer. Go back to the steering assist failure, if the motor or hydraulic pump gives up, a driver can still steer the vehicle, albeit with more effort on the steering wheel. Without that driver, an AV needs a secondary steering actuator to take over. The same goes for the brakes as well as power supplies to the compute, sensors, and other vehicle systems.
One of the reasons the Chrysler Pacifica Hybrid has become a common platform for many AV developers including Waymo, Voyage, Motional,
Aurora, and
AutoX is the availability of an AV-ready version. After Waymo went to
Fiat Chrysler in 2016 to purchase Pacificas for its test-fleet, FCA developed a version specifically for these applications that met the fail-operational requirements. These are produced on the same assembly line the minivans sold to consumers. New purpose-built robotaxis such as the one recently unveiled by Zoox and the Cruise Origin are also designed with similar capabilities.
One other nontrivial detail for an AV is the ability to keep the sensors clean. While the human brain has a remarkable ability to detect what is around the vehicle even when windows are dirty or otherwise obscured, the sensors need a clear view free of insects, bird droppings, and salt spray. Everyone that is serious about building AVs is also taking on this basic mechanical engineering and fluid dynamics problem.
IT TAKES MORE THAN A VEHICLE
Building a working AV is just the first step toward a successful robotaxi business. Behind every robotaxi is a whole lot of infrastructure including cloud computing, cleaning, charging, and maintenance operations. Modern ride-hailing services like Uber and Lyft are built on an asset-light model where the companies only provide a platform that connects drivers and passengers and takes a cut of every fare. They don’t have to worry about putting
fuel or electrons into the vehicle, maintaining wear items like washer fluids and
tires, or even cleaning the interiors.
However, regardless of whether the vehicles are owned by an individual with time on their hands, a fleet, or an automaker, all of these tasks and more are required to run a transportation business. As we make the transition from human-driven vehicles to robotaxis or automated delivery vehicles, a human will still be responsible.
INTO
THE CLOUD
All AVs are going to be wirelessly connected to infrastructure and likely to other vehicles. At a minimum, connectivity will be required to dispatch and summon vehicles. But these machines will rely on connectivity for so much more. AVs will inevitably encounter situations that they don’t know how to deal with, either because the software can’t understand something, or because it may require breaking some rules of the road.
In general, AVs will be designed to obey all the rules such as speed limits or where turns are allowed. But we’ve all encountered scenarios such as a double-parked delivery truck or impromptu construction zone or just a flood that may require a violation. It’s relatively straightforward for a human to make a judgment about when it’s okay to cross a double yellow line, make a U-turn, or go a few miles per hour over the speed limit. It’s much more difficult to program software to do that.
AVs will require some sort of remote operation or assistance system that feeds sensor data back to human supervisors in a control facility. Those operators can assist the AV about when it is safe to violate a rule or take an adjusted route. That will require high-speed data connections to stream sensor data. Most current development AVs have multiple bonded LTE connections to enable this. In the next year or so, vehicles will start transitioning from LTE to 5G (this is already happening in China).
These vehicles will also need regular updates for map and traffic information, as well as new software versions that improve functionality or correct security vulnerabilities. We definitely don’t want some bad actors to find a zero-day exploit that allows them to take control of a fleet of thousands or millions of AVs and tells them to instantly turn left and accelerate or perhaps come to a stop in a
ransom-ware attack.
All of this will require robust cloud services to manage the over-the-air software updates, aggregate data from AVs in the field for map updates, and collect sensor data from vehicles that have issues. These cloud services can also track when vehicles need to come in for fueling or charging as well as cleaning.
Scaling massive cloud infrastructure is no simple task as any growing internet-based service has learned over the years. Most current AV pilot programs are still relatively small scale but as they grow, these companies are likely to partner with one or more large cloud providers such as Amazon Web Services, Microsoft Azure, Google Cloud Platform,
Baidu or Alibaba in China.
Even before they get large-scale commercial deployments, massive amounts of data center storage and computing infrastructure are needed to ingest the multiple terabytes of raw data each vehicle collects daily. That data then needs to be parsed and labeled to train the neural networks that power these perception systems and run the billions of miles of simulations that are required just to validate the safety and capability of their AVs.
ONE
THE GROUND TASKS
Whether the drivers are human or digital and if the vehicle has passengers or packages, running a fleet efficiently is a complex task. Real estate isn’t usually top of mind for AV developers, but a fleet needs somewhere to call home. The power requirements of an ADS mean that these vehicles will all be electrified to various degrees. Some, like Ford’s upcoming AV launching in 2022 will use hybrid electric powertrains but they will still need to be fueled and serviced. Others such as
Waymo are using plug-in hybrid vehicles that will need some charging while the likes of Cruise and Zoox have developed purpose-built battery electric vehicles.
In addition to the energy supply, these vehicles will need replenishment of washer fluid to keep the aforementioned sensors clean, checking of tires and brakes, and just general cleaning and maintenance. A single robotaxi fleet in a city such as New York, London, or Shanghai will likely consist of at least several thousand vehicles and any such city will likely have multiple competing fleets. In addition to large scale depots, fleet operators will likely maintain smaller local depots around a city for fueling and quick cleaning. This distributed service network will help to minimize the amount of empty, deadhead miles the vehicles run during a workday and reduce overall downtime.
One of the major problems caused by individually owned and operated vehicles is limited utilization. A personal car is typically only used about 4% to 5% of the time and sits idle the rest of the day. That’s why the U.S. has more than seven parking spaces for every vehicle on the road. A shift to a shared, service-oriented transportation system can increase that utilization to more than 50% and in some cases to 75% or more.
This would help to make these more expensive vehicles commercially viable, but it will require all of the other parts of this mobility puzzle to be put in place.
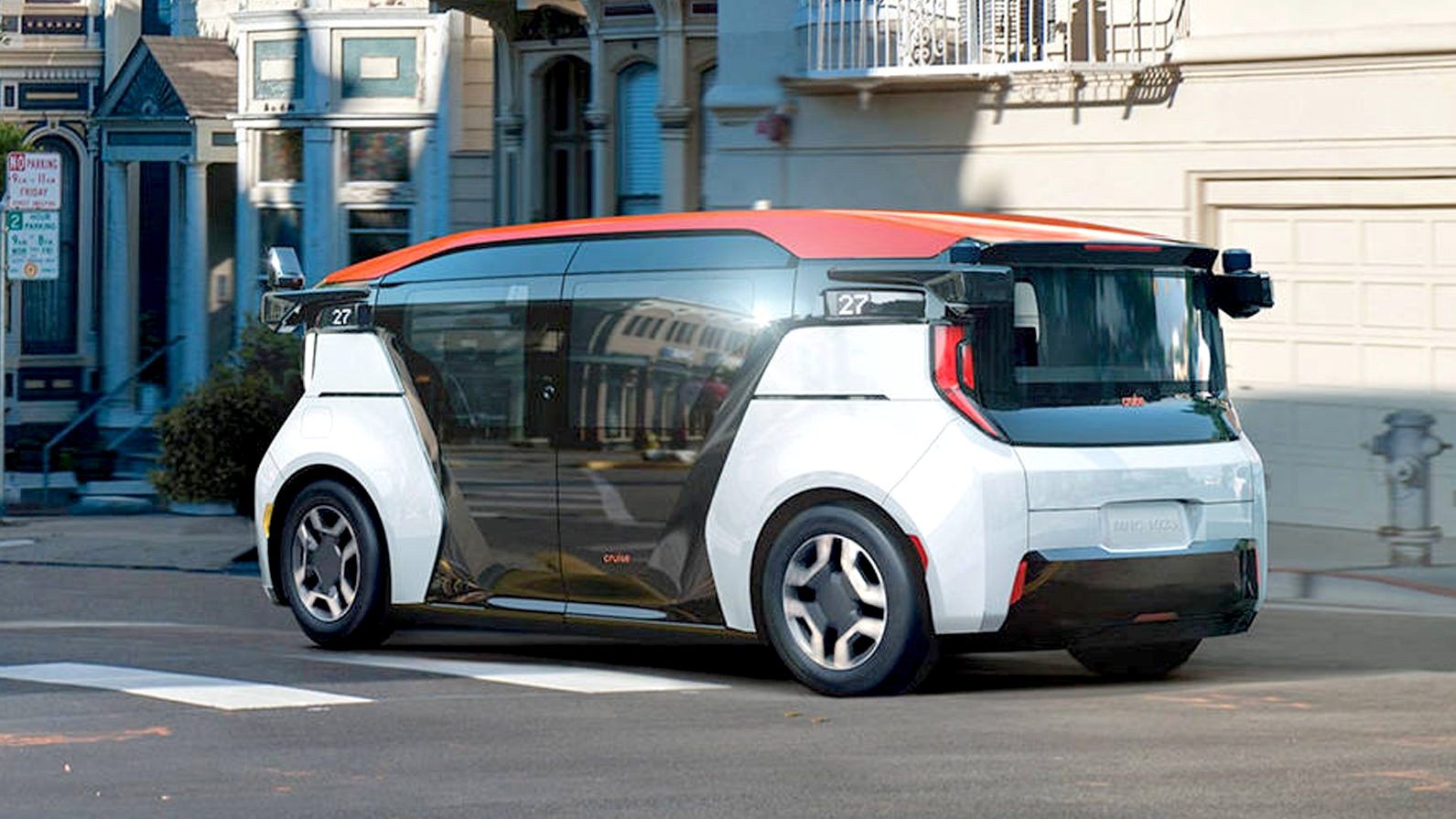
Added
to RoboCars, RoboTaxis
in cities, Robovans for goods deliveries, and RoboTrucks
for heavy haulage, it is possible that the face of transport is set to
change considerably in the years ahead. We anticipate that the price of
autonomous system components will level out, as chips, boards and sensors
reduce commensurate with anticipated production numbers.
Many
of these vehicles will need automated rapid battery recharging and hydrogen
refuelling, where they do not have drivers. At the moment, the only system
that would be capable of servicing such vehicles would be the proposed SmartNet™
dual fuel service stations, where such vehicles do not need human assistance
to replenish their energy reserves.
Allied
to this is PAYD
(Pay As You
Drive) billing, also seen as a necessary function for autonomous,
unmanned,
self-driving, robotic
vehicles of the future - in smarter cities.
LINKS
& REFERENCE
https://debugger.medium.com/an-automated-vehicle-expert-explains-how-close-we-are-to-robotaxis-d5ccc6c4c43d
UNMANNED ELECTRIC VEHICLES
THE AUTOMATED and ELECTRIC VEHICLES ACT 2018
Please
use our A-Z
INDEX to navigate this site
This
website is provided on a free basis to
promote zero emission transport from renewable energy in Europe and Internationally. Copyright ©
Universal Smart Batteries and Climate Change Trust 2021. Solar
Studios, BN271RF, United Kingdom.
|